
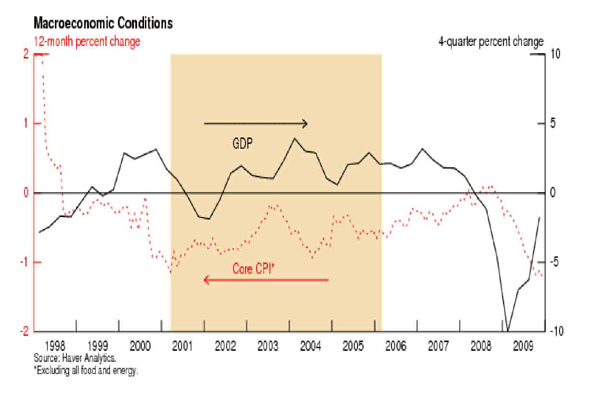
In these equations, predetermined and endogenous variables in level are instrumented with suitable lags of their own first differences. Thus, the original equations in levels can be added to the system, and the additional moment conditions could increase efficiency. This was created because lagged levels are often poor instruments for first differences, especially for variables that are close to a random walk. Alternatively, you may want to implement a system GMM model, the augmented version. You can implement a difference GMM model that treats the model as a system of equations, one for each time period, that differ only in their instrument/moment condition sets. The xtabond2 command offers you two opportunities. They both have one-step and two-step variants and the new command is now: xtabond2. Therefore, a new estimator commonly termed system GMM was implemented to substitute this basic one (known as difference GMM). His constructor Arellano later revealed a potential weakness of this estimator because the lagged levels are often rather poor instruments for first-differenced variables. The Stata command is xtabond, which basic syntax is: Tip: This estimator works well on datasets with many panels and few periods (N>T) and it requires that there is no autocorrelation in the idiosyncratic errors. The moment conditions are formed assuming that particular levels of the dependent variable are orthogonal to the differenced disturbances. This proposed method leads to consistent but not necessarily efficient estimates and is a variation of OLS in first differences model that uses an unbalanced set of instruments with further lags as instruments. Arellano and Bond suggested to use first differences to get rid of alphas and then using an IV method. If we use the first differences to get rid of alphas, OLS estimates remain inconsistent because of the lagged variable. If alphas are fixed-effects then the FE estimator is inconsistent. In this case, we have reasons to suppose that our dependent variable is serially correlated over time through its lag (true state dependence), through some covariates, which may be serially correlated (observed heterogeneity), or through alpha (unobserved heterogeneity). To see what I am talking about, let’s write down an AR(1) model with individual specific effects: Usually, simple dynamic model regresses a dependent variable in polynomial in time or on lags of itself, so they are characterized by the presence of a lagged dependent variable among the regressors. When do we face those data? Well, let’s say that many economic issues are dynamic by nature, like employment models.
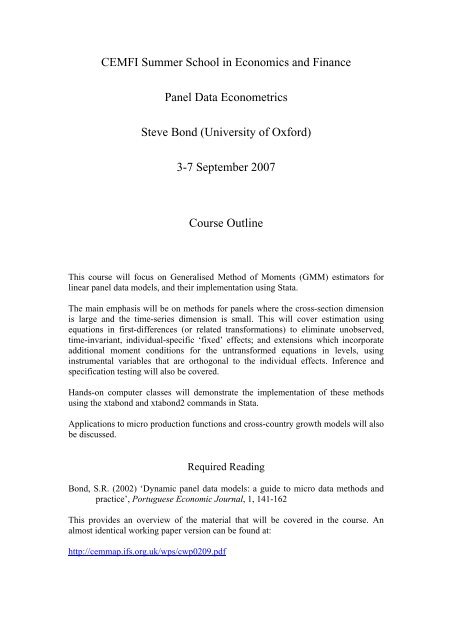
Today I want to spend some more words on Panel Data Analysis and extend our previous knowledge to what we know as Dynamic Panel data. All you have to know to use Panel Data proficiently using Stata
